Pillar 4: Data Transparency & Accountability
Contents
Pillar 4: Data Transparency & Accountability#
The attainment, usage, storage, analysis, and maintenance of human data should be as transparent, accountable, and honest as possible and intended for some sort of human benefit.
In order to facilitate reproducibility, trusted participation, and complete comprehension of terminal goals and results, a policy of transparency and honesty should be embedded into the protocol of handling human data. Transparency and honesty as it pertains to acquiring, using, storing, and analyzing data can include sharing information about these methods not only with stakeholders, but relevant government and regulatory agencies and the people that the data is collected from, when possible. Use of transparent and honest practices in each of these areas may increase the likelihood that the use of the data will in fact be beneficial to humans and may include, but are not limited to, the following practices:
Attainment - data was gathered through ethical and honest means, with the consent of subjects (See Pillar 2).
Usage - the use of data coincides with the intended use communicated to the subjects and does not include practices that maliciously targets groups of people or spreads misinformation (See Pillar 1).
Analysis - proper analytical methods are applied to garner understanding of data and does not include biased or inappropriate statistical applications, such as p-hacking, cherry picking, selective omission, and other problematic research practices1. This also includes conducting a meaningful cost-benefit analysis pertaining to accuracy vs. transparency.
Storage & Maintenance - secure means are used to preserve and house data physically or on an online server to ensure the privacy of subjects. Prior to collection, subjects are informed about what these privacy and security measures are (See Pillar 2).
Trust & Accountability#
The aforementioned practices may help to facilitate trust between human subjects/users and technologies that rely on data acquired from them 2, 3. The establishment of trust within communities can have several advantages, including increased use of a product or technology, expanded collection of data, improved productivity and efficiency in tasks, and other benefits 4. However, with the occurence of numerous malevolent incidences, including massive data breaches that revealed sensitive financial information 5 or the use of “synthetic” media that has contributed to social and political confusion and misinformation 6, it is unsurprising that some of the population has a level of distrust and concern when it comes to collection of personal data 7 and emergent technologies 8 such as artificial intelligence (AI), machine learning (ML), and natural language processing (NLP), in the public and private sectors.
In 2015, the Harvard Business Review published an article surveying consumers across several countries on sentiments around data privacy and security9. Interestingly, the entities people trusted most with their data were healthcare providers and financial institutions, while the least trusted were entertainment companies and social media firms. Arguably, the level of trust may coincide with mechanisms of accountability, such as HIPPA legislations and financial privacy laws like the Gramm–Leach–Bliley Act, which may not apply to entertainment and social media platforms in the same way. While legal obligation is a strong way to enforce accountability, providing open and accessible information on conduct around data security, privacy, and collection and protocols for when someone has questions or ailments about them shows an organization’s intrinsic commitment to transparent practices, which can enhance trust between the organization and customers, users, and other subjects from which data may be collected.
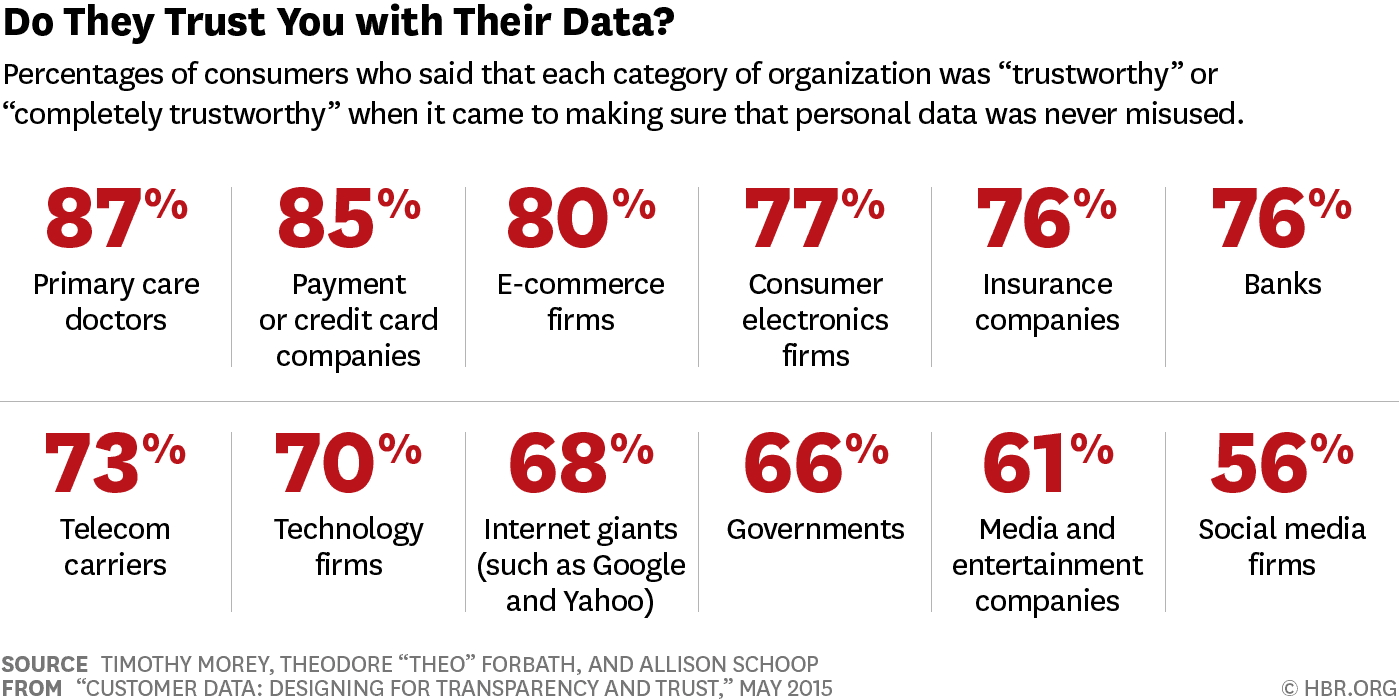
As laws relevant to companies outside of the healthcare and financial sectors are still developing, accountability for such entities is currently nebulous. The data lifecycle is a pipeline that considers the past, present, and future of data and its application in technologies by said companies. This pipeline involves numerous steps, such as data collection, processing, analysis, dissemination, and maintenance, which can be lead by numerous teams or organizations of people. Thus, for terminal applications of data, how do we determine accountability when things go wrong? While assignment of accountability is complex and multifaceted, Virginia Dignum, an AI ethicist and researcher, suggests that when thinking about accountability in the data lifecycle, the larger sociotechnical ecosystem must be considered within a Accountability-Responsibility-Transparency (ART) framework 10. Those involved throughout various phases of the data lifecycle should be able to explain and justify their decisions around data (accountability), acknowledge the role that they play in the data lifecyle (responsibility), and describe, inspect and reproduce mechanisms contributing to end products of the life cycle (transparency). Utilizing this framework requires a level of open discussion with stakeholders, whether they be clients, users, or society. Such discussions facilitate iterative modification and reworking to improve products and services from an ethical and technological standpoint.
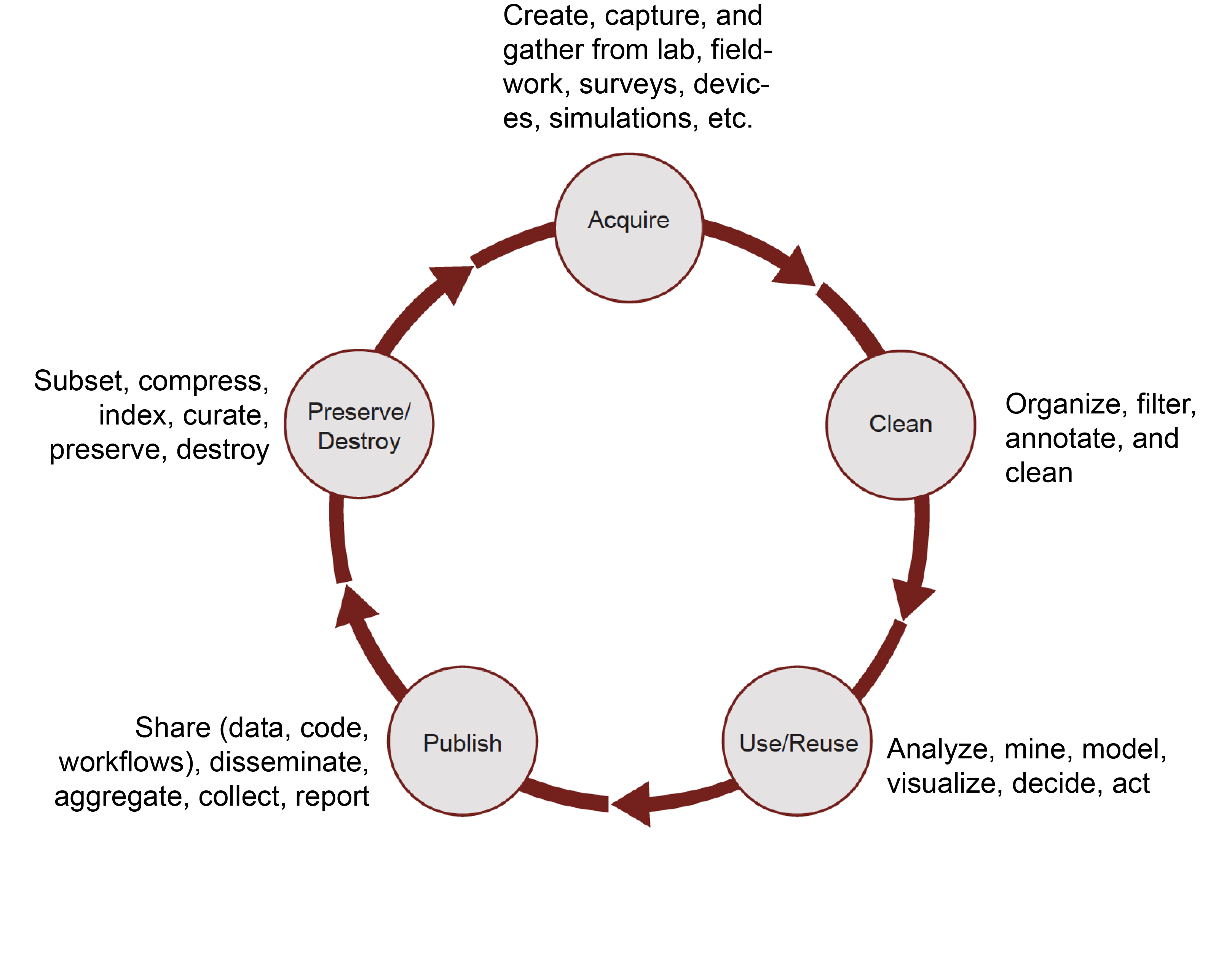
The Trade-Off Between Transparency and Accuracy#
The development of artificial intelligence algorithms has aided, informed, and/or influenced human decision-making processes in a number of low-stakes and high-stakes scenarios. Because of this, conversation around the transparency of these algorithms has highlighted important perspectives and viewpoints regarding the implications of these technologies.
Some algorithms disclose the use of parameters and models applied (i.e., “white-box” algorithms), while others may not readily explain the parameters and models used or may utilize another model that approximates the original model(s) (i.e., “black-box” algorithms). Some black-box algorithms also may utilize more sophisticated and complex methods, such as random forests and neural networks, which may not be readily explainable. Depending on the context of use, the methods backing white-box and black-box algorithms can influence the accuracy, and thus impact terminal services, decisions, products, or actions.
Explainable AI (XAI) and ML (XML) explores the intersection of transparency and technical applicability in AI and ML. While transparency can help promote accountability and trust, limitations to prioritizing transparency may present in the use of AI/ML. Some of these include malicious and unintended misuse of developed AI/ML tools 11, exposure of trade secrets 12, domain and technical knowledge requirements for full comprehension, and several others. In high-stakes situations, such as the use of AI/ML in healthcare decisions, some argue that accuracy should be more important than transparancy 13, while others suggest the use of interpretable models instead of black-box algorithms where possible 14. Sometimes, black-box algorithms can be replaced with more simpler and transparent ones with little compromise of accuracy 15. In cases where black-box methods must be used, attempts to provide transparency through justification as opposed to explanation may be the best case scenario 16. An evaluation of the situation and associated risks and rewards, as well as testing of multiple black-box, white-box, and interpretable options, is important in determining the the best way to balance transparency and accuracy.
Dishonest Statistical Practices#
A part of getting accurate insights from data includes using honest and appropriate statistical methods during data analysis. Doing such can enhance reproducibility, allowing for economical allocation of time and resources toward follow up studies. Some common pitfalls in research and analysis include hypothesizing after results are known (HARKing), p-hacking, and cherry-picking. Below is a discussion of each and how they impact research and knowledge generation.
HARKing#
As the acronym states, HARKing is developing a hypothesis about data after knowing the results that the data depict and then reporting conclusions as if they were hypothesized a priori. HARKing does not fully disclose the process leading to the hypothesis and conclusions from data and thus can be seen as dishonest in nature. HARKing may or may not include performing statistical analysis and determining significant variables in a dataset; plotting and cross-examining variables can also be a part of HARKing. When performing exploratory studies, examining the relationships between multiple variables from a dataset can be a useful process to generate new hypotheses, but these hypotheses should be tested with a new dataset to confirm previous observations.
P-hacking#
P-hacking can involve the use of multiple testing, various kinds of statistical tests, and/or specific subsetting of data in order to generate a significant p-value. Like HARKing, p-hacking does not fully account for the process leading up to a significant result. Analyzing data in various ways with the specific intent to show a significant p-value, rather than analyzing in an objective fashion, can lead to erroneous conclusions, misguided research directions, and retraction of scientific papers, as seen in the research of Dr. Brian Wansink 17. Because hypothesis testing reports the probability of an observed outcome occurring given that the null hypothesis is true, testing multiple hypothesis on the same data will give a false positive at some point. To address this, multiple testing corrections and adjustments should be used 18, 19.
Cherry-picking#
Cherry-picking involves biased selection of data for analysis or reporting conclusions. Cherry-picking can be used to fuel p-hacking or paint an incomplete picture of a research process. Reporting only data that aligns with a hypothesis can be an impediment to those trying to repeat a reported experiment because it can lead researchers down an avoidable rabbit hole. Furthermore, not reporting null data that is not in alignment with a hypothesis can similarly hinder the scientific process.
Data manipulation#
Data manipulation includes practices of fabrication and falsification that can be fueled by the omission, addition, and/or alteration of raw data. According to the National Science Foundation’s policy (45 CFR 689)20, fabrication means “making up data or results and recording or reporting them”, while falsification means “manipulating research materials, equipment, or processes, or changing or omitting data or results such that the research is not accurately represented in the research record.”
Data manipulation is one of the most blatantly dishonest research and statistical practices an investigator can do. It is greatly frowned upon within the scientific community and can reduce one’s credibility as a researcher, as seen with Dr. Francesca Gino21 and Dr. Dan Ariely.22 During the research process, it may be tempting to exclude perceived outliers within a dataset, but this should be avoided unless there is sound justification based on the data collection process or statistical backing; otherwise, keeping data points that deviate highly from others within the dataset is the most honest thing to do.
All-in-all, when it comes to data, especially when derived from humans, centering honesty as much as possible is the best policy.
- 1
Andrade, Chittaranjan. “HARKing, Cherry-Picking, P-Hacking, Fishing Expeditions, and Data Dredging and Mining as Questionable Research Practices.” The Journal of Clinical Psychiatry, vol. 82, no. 1, Feb. 2021, p. 20f13804, https://doi.org/10.4088/JCP.20f13804.
- 2
Lin, D., Crabtree, J., Dillo, I. et al. The TRUST Principles for digital repositories. Sci Data 7, 144 (2020). https://doi.org/10.1038/s41597-020-0486-7
- 3
Atske, Sara. “2. Americans Concerned, Feel Lack of Control over Personal Data Collected by Both Companies and the Government.” Pew Research Center: Internet, Science & Tech, 15 Nov. 2019, https://www.pewresearch.org/internet/2019/11/15/americans-concerned-feel-lack-of-control-over-personal-data-collected-by-both-companies-and-the-government/.
- 4
Robles, Pedro, and Daniel J. Mallinson. “Artificial Intelligence Technology, Public Trust, and Effective Governance.” Review of Policy Research, May 2023, p. ropr.12555, https://doi.org/10.1111/ropr.12555.
- 5
Mathews, Lee. “Equifax Data Breach Impacts 143 Million Americans.” Forbes, https://www.forbes.com/sites/leemathews/2017/09/07/equifax-data-breach-impacts-143-million-americans/. Accessed 31 July 2023.
- 6
U.S. Department of Homeland Security. Increasing Threat of DEEPFAKE Identities. Accessed 31 July 2023. https://www.dhs.gov/sites/default/files/publications/increasing_threats_of_deepfake_identities_0.pdf
- 7
“Americans Widely Distrust Facebook, TikTok and Instagram with Their Data, Poll Finds.” Washington Post, 22 Dec. 2021, https://www.washingtonpost.com/technology/2021/12/22/tech-trust-survey/.
- 8
Nadeem, Reem. “Public Awareness of Artificial Intelligence in Everyday Activities.” Pew Research Center Science & Society, 15 Feb. 2023, https://www.pewresearch.org/science/2023/02/15/public-awareness-of-artificial-intelligence-in-everyday-activities/.
- 9
Morey, Timothy, et al. “Customer Data: Designing for Transparency and Trust.” Harvard Business Review, 1 May 2015, https://hbr.org/2015/05/customer-data-designing-for-transparency-and-trust.
- 10
Virginia Dignum. The role and challenges of education for responsible AI. London Review of Education. 2021. Vol. 19(1). DOI: 10.14324/LRE.19.1.01
- 11
Umang Bhatt, Alice Xiang, Shubham Sharma, Adrian Weller, Ankur Taly, Yunhan Jia, Joydeep Ghosh, Ruchir Puri, José M. F. Moura, and Peter Eckersley. 2020. Explainable machine learning in deployment. In Proceedings of the 2020 Conference on Fairness, Accountability, and Transparency (FAT* ‘20). Association for Computing Machinery, New York, NY, USA, 648–657. https://doi.org/10.1145/3351095.3375624
- 12
Burrell, J. (2016). How the machine ‘thinks’: Understanding opacity in machine learning algorithms. Big Data & Society, 3(1). https://doi.org/10.1177/2053951715622512
- 13
Ghassemi M, Oakden-Rayner L, Beam AL. The false hope of current approaches to explainable artificial intelligence in health care. Lancet Digit Health. 2021 Nov;3(11):e745-e750. doi: 10.1016/S2589-7500(21)00208-9. PMID: 34711379.
- 14
Rudin C. Stop Explaining Black Box Machine Learning Models for High Stakes Decisions and Use Interpretable Models Instead. Nat Mach Intell. 2019 May;1(5):206-215. doi: 10.1038/s42256-019-0048-x. Epub 2019 May 13. PMID: 35603010; PMCID: PMC9122117.
- 15
Chaofan Chen, Oscar Li, Chaofan Tao, Alina Jade Barnett, Jonathan Su, and Cynthia Rudin. 2019. This looks like that: deep learning for interpretable image recognition. Proceedings of the 33rd International Conference on Neural Information Processing Systems. Curran Associates Inc., Red Hook, NY, USA, Article 801, 8930–8941, and Barnett, A.J., Schwartz, F.R., Tao, C. et al. A case-based interpretable deep learning model for classification of mass lesions in digital mammography. Nat Mach Intell 3, 1061–1070 (2021). https://doi.org/10.1038/s42256-021-00423-x
- 16
Biran, Or and Courtenay V. Cotton. “Explanation and Justification in Machine Learning : A Survey Or.” (2017).
- 17
“More Evidence That Nutrition Studies Don’t Always Add Up.” The New York Times, 29 Sept. 2018, https://www.nytimes.com/2018/09/29/sunday-review/cornell-food-scientist-wansink-misconduct.html.
- 18
Benjamini, Yoav, and Yosef Hochberg. “Controlling the False Discovery Rate: A Practical and Powerful Approach to Multiple Testing.” Journal of the Royal Statistical Society. Series B (Methodological), vol. 57, no. 1, 1995, pp. 289–300. JSTOR, http://www.jstor.org/stable/2346101. Accessed 22 Aug. 2023.
- 19
Zbyněk Šidák (1967) Rectangular Confidence Regions for the Means of Multivariate Normal Distributions, Journal of the American Statistical Association, 62:318, 626-633, DOI: 10.1080/01621459.1967.10482935
- 20
Code of Federal Regulations. Title 45, Part 689 - Research Misconduct. 18 Mar. 2002. https://www.ecfr.gov/current/title-45/subtitle-B/chapter-VI/part-689.
- 21
“Professor accused of faking data in studies on dishonesty sues Harvard.” Washington Post, 3 Aug. 2023, https://www.washingtonpost.com/education/2023/08/03/harvard-honesty-lawsuit-research-misconduct/.
- 22
“An Influential Study Of Dishonesty Was Dishonest.” Forbes, 30 Aug. 2021. https://www.forbes.com/sites/christianmiller/2021/08/30/an-influential-study-of-dishonesty-was-dishonest/?sh=6e9e992c2c72.